What Generative AI Means for Cybersecurity in 2024
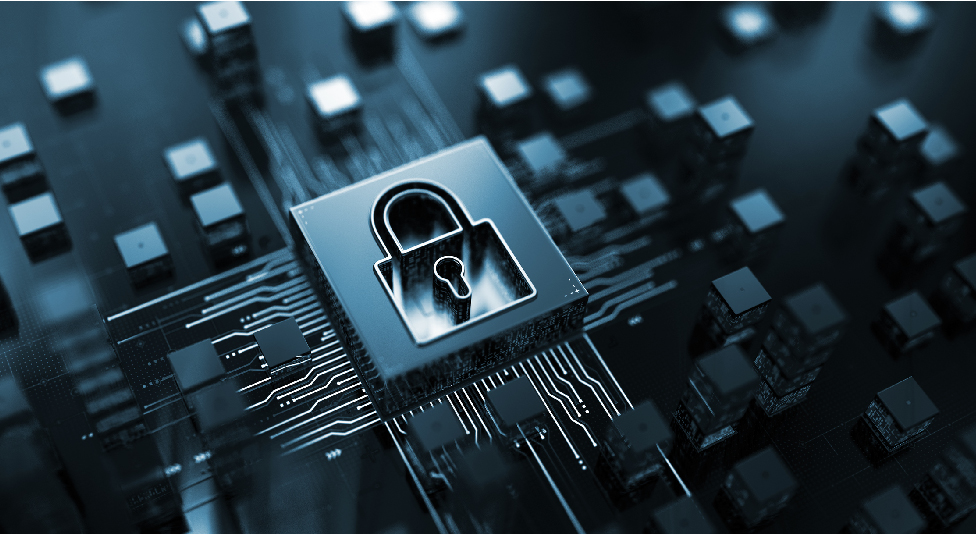
(Mis)using generative AI for cybercrime
When public versions of generative AI first hit the scene, some experts worried criminals would create dark GPT models and other kinds of generative AI engines to produce ‘unstoppable’ malware. There’s definitely demand for this, and attempts have been made, but so far it appears easier said than done. FraudGPT, which garnered attention in 2023, seems to have been basically vaporware for criminals—just promotional and demo material—and the WormGPT tool that captured headlines toward the end of the year was shelved in days due to the media attention it received.
Online discussions on criminal forums about “How to Create Your Own Malicious GPT” are largely focused on tips and tricks to leverage existing LLM infrastructure (i.e. LLaMA) to their advantage.Today, developing a purpose-built criminal large language model (LLM) may be too costly and labor-intensive for bad actors compared to jailbreaking publicly available AI apps—exploiting weaknesses in their rules or constraints to generate outputs that go against intended uses.
That said, nefarious LLM development efforts are likely to persist in 2024, accompanied by new tools for malware authorship and other tasks. As information theft increases, a whole new cybercriminal service—‘reconnaissance as a service’ (ReconaaS)—is likely to emerge. Certain bad actors will use AI to extract useful personal information from stolen data and sell it to other cybercriminals for ultra-targeted attacks.
Generative AI has already accelerated the race to discover vulnerabilities in open-source software by making it possible to compare different software versions’ source code and find not just disclosed vulnerabilities but undisclosed ones as well.
As noted, criminals are also targeting AI apps themselves. The earliest attempts to do so involved injecting malicious prompts into AI systems to make them misbehave, but these have proved relatively east to combat by not training public AI tools on user inputs. More recently, hijacking and jailbreaking apps have become trending topics in cybercrime forums, indicating high criminal interest. These tactics are likely to gain ground in 2024.
Evolving defense strategies to match gen AI threats
Despite the AI-enabled intensification of cybercrime, wins are available to defenders as long as organizations are prepared to adapt. What’s needed is a combination of zero-trust approaches and the use of AI to make security stronger.
As the name implies, with zero trust, trust is never presumed. Identities must always be verified, and only necessary people and machines can access sensitive information or processes for defined purposes at specific times. This limits the attack surface and slows attackers down.
Applied to the earlier example of the phony purchase order email with deepfake voice confirmation, zero-trust verification would prohibit users from calling the number in the message. Instead, they would have an established ‘safe list’ of numbers to call, and/or need multi-stakeholder approval to verify the transaction. Coded language could even be used for additional authentication.
Even though phishing attacks are now too well disguised for users to detect them on their own, cybersecurity awareness training remains essential; it just needs to be backed up with defensive technologies. AI and machine learning can be used to detect sentiment and tone in messages or evaluate web pages to prevent fraud attempts that might slip by users.
Harnessing generative AI for good
Generative AI can help cybersecurity teams work faster and be more productive by providing plain-language explanations of alerts, decoding scripts and commands, and enabling precise and effective search queries for analysts who aren’t specialized in search languages. It acts as a ‘force multiplier’ by automatically enacting security response playbooks as soon as incidents occur.
AI-driven automation can also eliminate the burden of incident reporting, which is key for regulated industries: handling ticketing and reporting, translating reports into multiple languages, and extracting actionable information from documentation at high speed.
Remediation and response can both be strengthened when generative AI is used for comprehensive risk prioritization and to produce customized risk reduction and threat response recommendations. It can even identify which AI apps users are working with—and where, and how.
Since it’s unrealistic to ban AI apps outright, organizations need to be able to manage them. And for their part, developers need to prioritize safety and anti-abuse as they’re creating them.
The benefits of generative AI multiply with deep integration into cybersecurity platforms such as extended detection and response (XDR) that provide cross-vector telemetry from endpoints to the cloud.
Finally, generative AI can help enhance proactive cyber defenses by enabling dynamic, customized, industry-specific breach and attack simulations. While formalized ‘red teaming’ has typically been available only to the biggest organizations with deep pockets, generative AI has the potential to democratize the practice by allowing organizations of any size to run dynamic, adaptable event playbooks drawing from a wide range of techniques.
Making generative AI part of a healthy cybersecurity diet
Cybercriminals will use generative AI to their advantage however they can. The past year has shown they have the will, if not always the way—yet. But generative AI coupled with zero-trust security frameworks, adaptive practices, and security-aware organizational cultures also equips organizations to mount a strong and proactive defense.
Read More HERE