How to Write a Generative AI Cybersecurity Policy
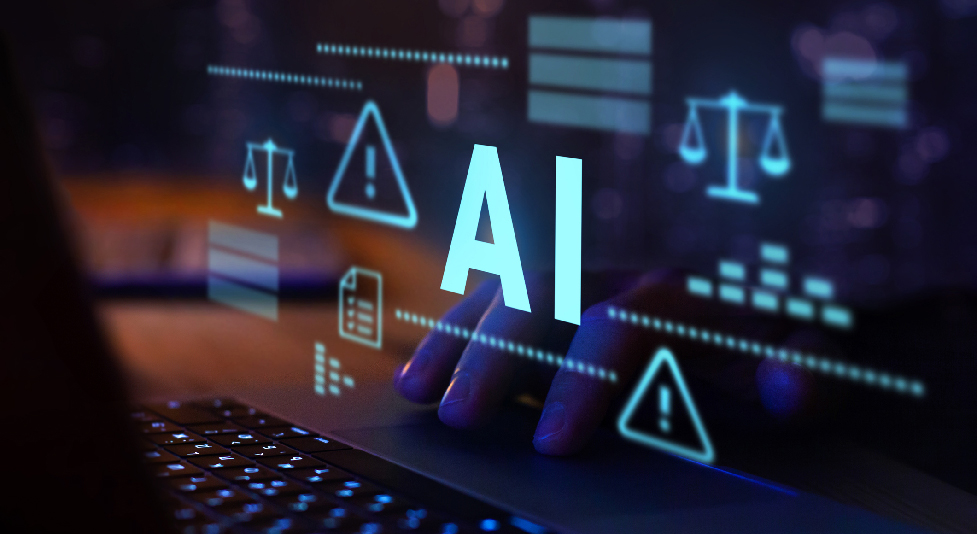
Amidst all the hype, CISOs urgently need practical guidance on how to establish AI security practices to defend their organizations as they play catchup with deployments and plans. With the right combination of cybersecurity policy and advanced tools, enterprises can meet their goals for today and lay a foundation for dealing with the evolving complexities of AI going forward.
When the best and brightest people working on a new technology say mitigating its risks should be a global priority, it’s probably wise to pay attention. That famously happened on May 30, 2023, when the Center for AI Safety published an open letter signed by more than 350 scientists and business leaders warning of the most extreme potential dangers posed by AI.
As much of the ensuing media coverage pointed out, fearing the hypothetical absolute worst may actually be a dangerous distraction from confronting AI risks we already face today, such as internal bias and made-up facts. The last of these made headlines recently when one lawyer’s AI-generated legal brief was found to contain completely fabricated cases.
Our other AI blogs have looked at some of the immediate AI security risks corporate CISOs should be thinking about: the ability of AI to impersonate humans and perpetrate sophisticated phishing schemes; lack of clarity about the ownership of data entered into and generated out of public AI platforms; and outright unreliability—which includes not just bad information created by AI but also AI ‘poisoned’ by bad information it absorbs from the Internet and other sources.
I’ve argued in chats with ChatGPT about facts concerning network security after getting incorrect information, and forcing it to disclose the correct answer it seemed to know all along. Whereas ChatGPT lists as a feature for their Enterprise version that no training will be done on your data, of course not all employees and contractors will use only an Enterprise version. And even if a private language AI instance is to be used, the impact of a breach of any AI whether public or private bears consideration.
If those are the risks, the next obvious question is, “What can CISOs do to boost their organizations’ AI security?”
Good policy is the foundation of AI security
Corporate IT security leaders learned the hard way over the past decade that prohibiting the use of certain software and devices typically backfires and can even increase risk to the enterprise. If an app or solution is convenient enough—or if what’s sanctioned by the company doesn’t do everything users need or want—people find a way to stick with the tools they prefer, leading to the problem of shadow IT.
Considering that ChatGPT snapped up more than 100 million users within just two months of launching, other generative AI platforms are already well embedded into people’s workflows as well. Banning them from the business could create a ‘shadow AI’ problem more perilous than any sneak-around solution that has come before. Also, many corporations are driving AI adoption as a way to boost productivity and would now find it difficult to block its use. If the policy decision is to ban unapproved AI, there must be detection and possibly blocking.
What CISOs need to do, then, is give people access to AI tools supported by sensible policies on how to use them. Examples of such policies are starting to circulate online for large language models like ChatGPT, along with advice on how to evaluate AI security risks. But there are no standard approaches as of yet. Even the IEEE doesn’t have its arms fully around the issue, and while the quality of information online is steadily improving, it is not consistently reliable. Any organization looking for AI security policy models should be highly selective.
Four key AI security policy considerations
Given the nature of the risks outlined above, protecting the privacy and integrity of corporate data are obvious goals for AI security. As a result, any corporate policy should, at a minimum:
1. Prohibit sharing sensitive or private information with public AI platforms or third-party solutions outside the control of the enterprise. “Until there is further clarity, enterprises should instruct all employees who use ChatGPT and other public generative AI tools to treat the information they share as if they were posting it on a public site or social platform,” is how Gartner recently put it.
2. Don’t “cross the streams”. Maintain clear rules of separation for different kinds of data, so that personally identifiable information and anything subject to legal or regulatory protection is never combined with data that can be shared with the public. This may require establishing a classification scheme for corporate data if one doesn’t already exist.
3. Validate or fact-check any information generated by an AI platform to confirm it is true and accurate. The risk to an enterprise of going public with AI outputs that are patently false is enormous, both reputationally and financially. Platforms that can generate citations and footnotes should be required to do so, and those references should be checked. Otherwise, any claims made in a piece of AI-generated text should be vetted before the content is used. “Although [ChatGPT] gives the illusion of performing complex tasks, it has no knowledge of the underlying concepts,” cautions Gartner. “It simply makes predictions.”
4. Adopt—and adapt—a zero trust posture. Zero trust is a robust way of managing the risks associated with user, device, and application access to enterprise IT resources and data. The concept has gained traction as organizations have scrambled to deal with the dissolution of traditional enterprise network boundaries. While the ability of AI to mimic trusted entities will likely challenge zero-trust architectures, if anything, that makes controlling untrusted connections even more important. The emerging threats presented by AI make the vigilance of zero trust critical.
Choosing the right tools
AI security policies can be backed up and enforced with technology. New AI tools are being developed to help spot AI-generated scams and schemes, plagiarized text, and other misuses. These will eventually be deployed to monitor network activity, acting almost as radar guns or red light cameras to spot malicious AI activity.
Already today, extended detection and response (XDR) solutions can be used to watch for abnormal behaviors in the enterprise IT environment. XDR uses AI and machine learning to process massive volumes of telemetry (i.e., remotely gathered) data to police network norms at volume. While not a creative, generative type of AI like ChatGPT, XDR is a trained tool that can perform specific security tasks with high precision and reliability.
Other types of monitoring tools such as security information and event management (SIEM), application firewalls, and data loss prevention solutions can also be used to manage users’ web browsing and software use, and to monitor information leaving the company IT environment—minimizing risks and potential data loss.
Know your limits
Beyond defining smart corporate policies for AI security and making full use of current and novel tools as they emerge, organizations should get specific about the degree of risk they’re willing to tolerate to take advantage of AI capabilities. An article published by the Society for Human Resource Management recommends that organizations formally determine their risk tolerance to help make decisions about how extensively AI can be used—and for what.
The AI story has barely begun to be written, and no one has a sure grasp on what the future holds. What’s clear is that AI is here to stay and, despite its risks, has much to offer if we build and use it wisely. Going forward, we’ll increasingly see AI itself deployed to fight malicious uses of AI, but for now the best defense is to start with a thoughtful and clear-eyed approach.
Further insights
For more Trend Micro thought leadership on AI security, check out these resources:
Read More HERE